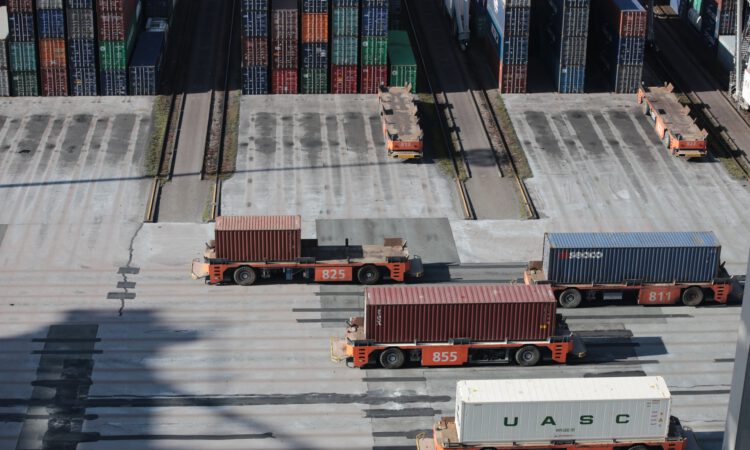
Powerhouse teams through the U.S., Germany, Japan and in other places flew into teach, participate and prove on their own a worldwide.
They’re maybe not competing in quadrennial games in Rio de Janeiro, nevertheless Amazon selecting Challenge, presented this present year at Robocup 2016 in Leipzig, Germany. Now with its second year, the event challenges autonomous robots to figure out how to select and stow items.
Your competition marked a bellwether minute for GPU-powered deep understanding in warehouse and factory automation, whilst played an essential part in five of the 10 top ranking teams, such as the top two finishers.
Deep discovering “neural nets played a big component in several teams’ methods to object recognition this year, a significant vary from last year, ” stated Joey Durham, supervisor of analysis and Advanced developing at Amazon Robotics.
The Future of Warehouse Automation
Amazon has actually made use of logistics robots in its vast warehouses across the globe to maneuver around shelving devices and minimize the hunt for things it’s going to provide to its millions of clients. But identifying and retrieving things for packaging, shipment and stowing however requires a lot of work manually.
To automate these jobs and develop links between your manufacturing and educational robotics communities, Amazon created the Amazon choosing Challenge. Participating teams tend to be challenged to deploy robots that will autonomously recognize things and choose, and stow, the specified objectives from a range of unsorted products.
Netherlands’ TU Delft Takes First Place for Stowing and Selecting
The winning team when it comes to separate picking and stowing difficulties was Delft University of Technology (TU Delft) in Netherlands, doing work in collaboration with Delft Robotics, an affiliated startup. The group surely could identify objects in only 150 milliseconds.
TU Delft used an NVIDIA TITAN X GPU and about 20, 000 images to teach a “base” model, where they built designs both for a “bin” and a “tote” arrangement. They taught their particular deep understanding system using PyFaster-RCNN, which utilizes the Caffe framework and it is hardware-accelerated by NVIDIA cuDNN.
“After these results, we at Delft Robotics are currently working on implementing the knowledge of GPU computing that we acquired during the challenge and deploying these algorithms in industrial systems, ” said Hans Gaiser, computer vision programmer at Delft Robotics.
Germany’s UBonn Snatches 2nd Place for Stowing
Team NimbRo from University of Bonn took 2nd invest the stowing challenge. The team integrated depth quotes from infrared light projection as well as 2 Intel RealSense SR300 level cameras. It also sized thick stereo level from two incorporated full-HD shade cameras.
Team NimbRo’s method incorporated education regarding ImageNet challenge, a well known image recognition standard, and annotation on a comparatively few pictures for the Amazon selecting Challenge scenes. For education and recall, it used a Supermicro GPU workstation with four NVIDIA GeForce GTX TITAN X GPUs.
Japan’s PFN Grabs 2nd Place for Selecting
Japan’s popular systems, collaborating with FANUC, an industrial automation giant, came in second devote the choosing challenge. The utilized convolutional neural networks for two various tasks: picture segmentation making use of RGBD information made by an Intel RealSense SR300 camera and an NVIDIA GeForce GTX 870M notebook GPU to do segmentation at 0.1 moments per picture, in place of 2 seconds per picture on a CPU.